Gergely Flamich
Imperial College Research Fellow, Imperial College London. Curriculum Vitae
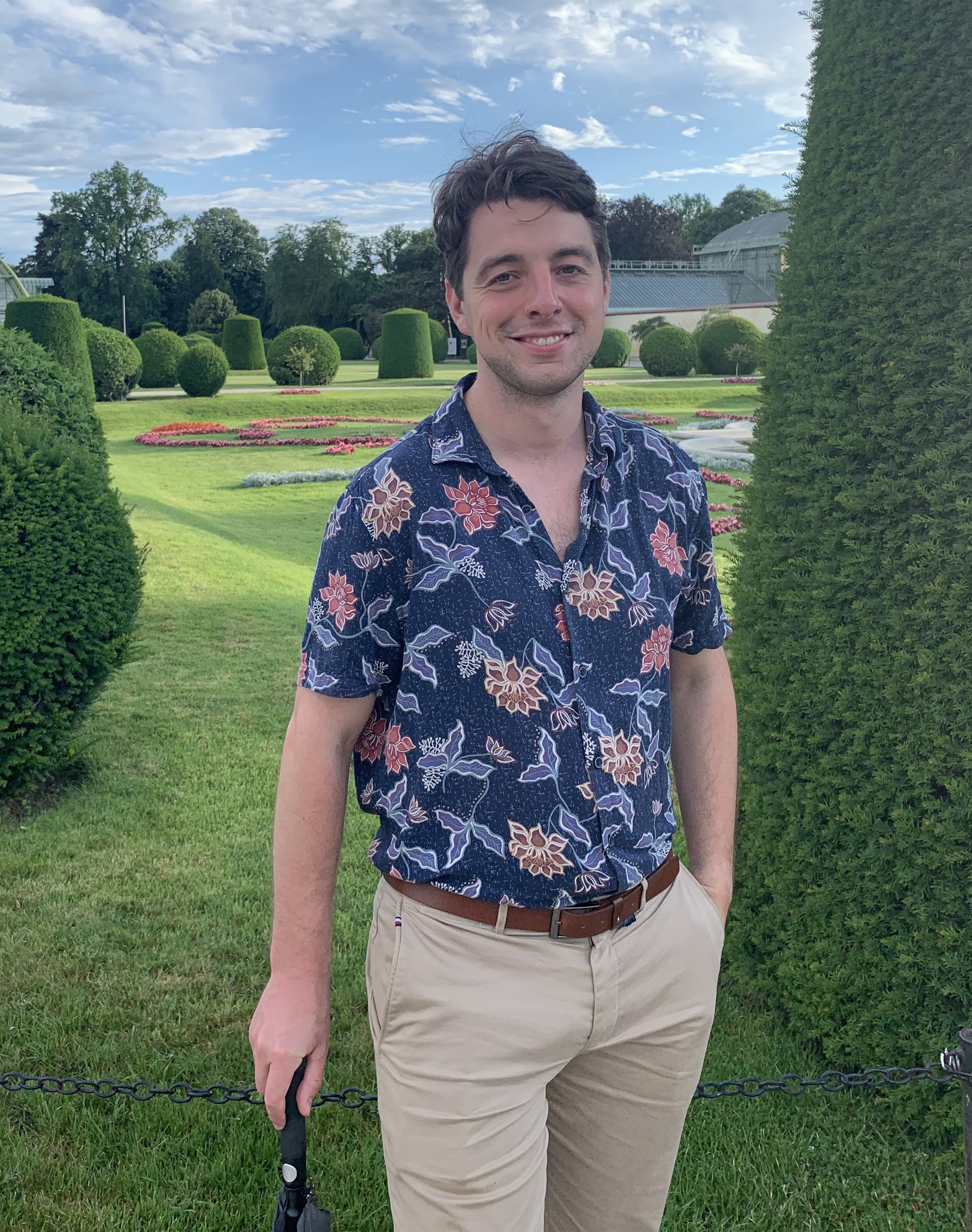
Information Processing and Communications Lab,
Department of Electrical and Electronic Engineering,
Imperial College London,
South Kensington Campus, London, UK
Hello there! I’m Gergely Flamich (pronounced gher - gey flah - mih but in English, I usually go by Greg), and I’m originally from Vác, Hungary.
From 1 August 2025, I am an Imperial College Research Fellow, sponsored by Deniz Gündüz and hosted at the Information Processing and Communications Lab at Imperial College London. Before that, I spent five months as a postdoctoral research associate in the same lab.
I completed my PhD degree between October 2020 and December 2024 in Advanced Machine Learning at the Computational and Biological Learning Lab, supervised by José Miguel Hernández Lobato. I also hold an MPhil degree in Machine Learning and Machine Intelligence from the University of Cambridge and a Joint BSc Honours degree in Mathematics and Computer Science from the University of St Andrews.
During the winter of 2024-2025, I worked with David Vilar, Jan-Thorsten Peter and Markus Freitag as a Student Researcher at Google Berlin. We worked on generative models for neural machine translation and we developed the theory of the accuracy-naturalness tradeoff in translation.
From July 2022 until Dec 2022, I worked with Lucas Theis as a Student Researcher at Google Brain, during which time we developed adaptive greedy rejection sampling and bits-back quantization.
My research focuses on the theory of relative entropy coding/channel simulation and its application to neural data compression. Relative entropy coding algorithms allow us to efficiently encode a random sample from both discrete and continuous distributions, and they are a natural alternative to quantization and entropy coding in lossy compression codecs. Furthermore, they bring unique advantages to lossy compression once we go beyond the standard rate-distortion framework: they allow us to design optimally efficient artefact-free/perfectly realistic lossy compression codecs using generative models and perform differentially private federated learning with optimal communication cost. Unfortunately, relative entropy coding hasn’t seen widespread adoption, as all current algorithms are either too slow or have limited applicability.
Hence, I am focusing on addressing this issue by developing fast, general-purpose coding algorithms, such as A* coding and greedy Poisson rejection sampling, and providing mathematical guarantees on their coding efficiency and runtime. In addition to my theoretical work, I am also interested in applying relative entropy coding algorithms to neural compression, utilizing generative models such as variational autoencoders and implicit neural representations.
Judit, my wonderful sister, is an amazing painter. Check out her work on Instagram!